July 13, 2021
Cases and examples on how to conduct an Ethical AI Risk Assessment

In our last blogpost, we have talked about what Trustworthy and Ethical AI is, and how we at ML6 help our clients build trust with their customers and employees through our Ethical AI Risk Assessment. In this post, we want to make it more practical, and demonstrate which dimensions and questions we might consider during such an assessment.
Conducting an Ethical AI Risk Assessment in practice
The need for building Trustworthy AI is clear (if you don’t know yet what we mean by Trustworthy AI, check out this blog post or this video). But how do you approach reviewing AI solutions in practice? Let’s go through a hypothetical example together, using the concept detailed in the last blogpost.
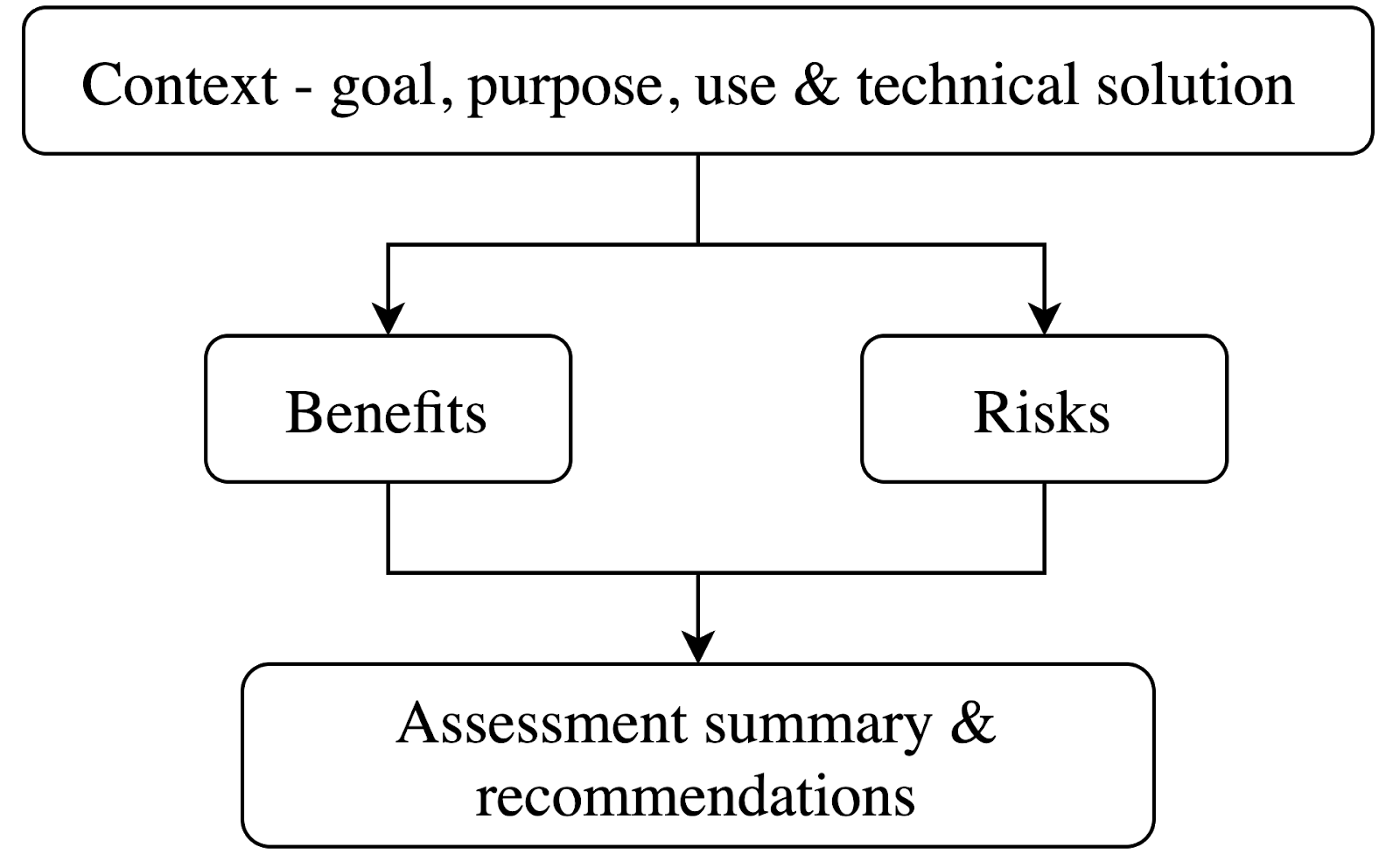
In the beginning of the Corona crisis, a few of our ML6 agents worked on a proof of concept to detect (missing) face masks on a camera feed with computer vision to keep our office safe (see this blog post). We’ll use that project as our example. In people-focused projects we typically want to pay even more attention to ethical concerns - so the face mask detection is an ideal case in point.
Let’s be clear - the solution we will be describing and assessing is not actually used in any of our offices. But for the sake of demonstrating how to practically go about an Ethical Risk assessment, let’s assume that we would want to actually implement the proof of concept.
Goal, context & technology
Let’s dive right in. We first need to get an overview of the project - what is the goal and purpose of the solution? How is it technically built and in which context does it operate? In our example, our goal would be to use the face mask detection solution to help our offices monitor whether Covid-19 safety measures are being respected. The solution would check with computer vision whether people wear a face mask when entering the office building and, if a missing mask is detected, give a verbal alert. From a technical perspective, the (experimental) Proof of Concept solution uses OpenCV to stream, process and modify camera images. We developed a first model using MTCNN, and subsequently trained our own Tiny YOLO model for detecting the person’s mouth for each detected face - assuming that if we can see a person’s mouth, the person is not wearing a face mask (for more technical details, check out the original blog post). Lastly, we look at the context. The mouth mask detector was built in the context of the global health crisis, during which many countries have passed regulations to wear face masks in public places. As you might notice, the context in this case is especially important - there would be no reason or benefit to implement such a solution outside of the current health crisis.
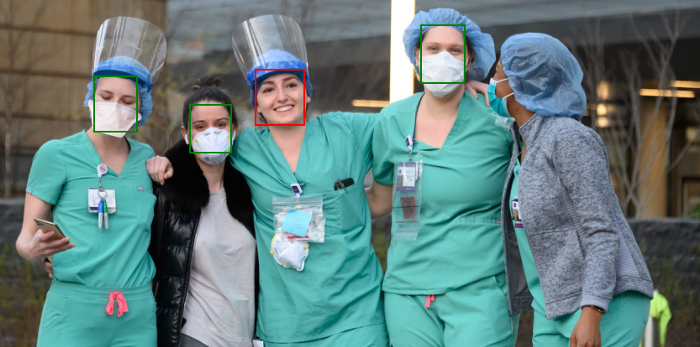
Benefits & Risks
Once we have a detailed overview of the solution, we need to assess its benefits and risks. On the benefits side, we consider three levels - benefits for the organisation, the individual and society as a whole. For our organisation, benefits include making sure that employees (or customers) feel safe and carry less risk of falling ill, as well as less effort to do manual controls. For the individual, similar benefits come to mind - easy reminders, a safer office environment and fewer awkward conversations asking your coworkers to put on a mask. For society, any measure that provides a small contribution to ultimately contain the health crisis might bring benefits. Of course the realization of these benefits strongly depends on the actual use of the solution, the acceptance and compliance with its suggestions, and many other factors.
Let’s continue with the risk side. Here we follow the 7 risk dimensions as defined by the EU guidelines for Trustworthy AI, and identify the risks by answering a curated set of questions.

For demonstration purposes, we listed a few sample questions for each dimension here, but rest assured that we would look at many more questions in a real-life assessment.
1. Human agency and oversight:
In this dimension, we look at risks related to the influence AI can have on human behaviour and decision making. For example, we should ask questions such as:
- Is there a human in the loop that verifies predictions and oversees the solution?
- Could humans develop a blind trust for automation (that is, assuming that the machine is always right)?
- Is there a risk that the user could think he’s interacting with another human, not an AI solution?
For our example, this dimension carries rather limited risk. Even if there is indeed no human in the loop that verifies each prediction, there seems to also be limited harm that can be done when a prediction is not correct - in the worst case, we are back to reminders by your coworkers (to give a counterexample, this would be very different for an AI solution that predicts for example medical treatment options for a patient - we would want each of these predictions to be verified by a qualified doctor). Our employees are also unlikely to blindly trust the machine, and we are confident that everyone would know the interaction is with a machine - the mechanical voice clearly gives this away.
2. Technical robustness and safety:
AI systems need to be resilient, secure, safe and reliable, in order to prevent potential harm. In this dimension we answer questions such as:
- Are users aware how accurate the solution is?
- Could there be any damages (e.g. to safety) if the system stops working or if there are technical defects?
- What could an actor with bad intentions do with the solution?
Technical robustness and safety will be a higher risk dimension in our case. Some damages, in this case to the health of employees, might occur if the solution stops working, however we believe that most people would become aware of the missing mask rather quickly. More concerning is the fact that it’s quite easy to trick the solution. If you remember the beginning of the assessment, we explained that we’re actually detecting missing mouths, not the presence of masks - so how about hiding your mouth behind your hand? We might have to build a more robust solution if this turns out to be a problem. Of course this concern is even more important for AI that could cause greater harm, think for example of self-driving cars. You would want the technology to be as robust and reliable as possible (... is your car able to correctly recognize street signs if they are covered by graffiti?)
The highest risk in this dimension though, not related to AI, is that a bad actor could take control of the camera or misuse the solution to spy on employees.
3. Privacy and data governance:
Privacy is a fundamental right particularly affected by AI systems. A solution needs to comply with legal requirements (e.g., GDPR), but should also go a step further and include the ethical perspective.
- Can impacted individuals object to the collection of their data?
- Could you work with anonymized or pseudonymised data instead?
- What is the worst headline in a newspaper related to privacy breaches you could imagine for this project?
The privacy dimension, as could be expected, likely carries the highest risks in this particular case. We are using facial recognition to identify faces and facial features, which is a highly controversial topic. However, the application will not store any data and is purely executed on edge, making it less prone to misuse or attacks.
Note: from a legal perspective, a Data Processing Impact Assessment (Art. 35 GDPR) will be needed to identify, manage and mitigate the privacy risks from a legal and technical perspective as well.
4. Transparency and explainability:
Explainability of an AI solution is an important aspect for Ethical AI. Not only do we want to be able to explain which features have been considered by a model & how the model works, but explainability is also needed to ensure that we can trust the model, and might even bring additional insights. Questions we might ask ourselves are:
- How much of the data sources can you disclose?
- Can you easily explain the model and its decisions to (non-technical) users?
- Can you transparently explain & communicate to the people impacted by the AI? If not, is there a good reason why not?
The solution we have built is quite transparent - all data, outputs and models used are publicly communicated and available. While the use case itself is quite easy to understand, the underlying models however might be less clear to non-technical users. For example, the Yolo model used could be more difficult to understand, being a quite complicated convolutional neural network architecture trained on a large amount of data.
5. Diversity, non-bias and fairness:
Bias or incompleteness of the data sets used by AI systems or of the system itself might lead to unfairness or cause harm. We need to actively identify potential risks of bias and mitigate them to build fair, non-biased solutions. Questions we might ask ourselves:
- Can you think of a group of people that is under- or overrepresented in the data set?
- Whose perspective is missing in the development and design process?
- What could be a potential historical bias in the data you are using?
The dimension of diversity and fairness could be high risk in our case. We would need to have a close look at the training data set - could there be a group of people that is underrepresented in the training data, for example ethnic minorities? If so, it is imaginable that the faces of these groups are detected with lower accuracy. Such issues might especially go unnoticed if our development and testing team did not include a diverse enough set of people.
6. Environmental and societal wellbeing:
The broader impact of AI on society and the environment should be considered as well of course. We could think about the following:
- How would your product change if you focus on building the most environmentally friendly solution you can?
- Might any jobs be at stake as a consequence of implementing the solution?
- What is the potential negative impact of the solution on society or democracy?
At ML6, we have strong principles on developing sustainable solutions. The use of pretrained open-source models with optimized model size here for example helps with building as environmentally efficient solutions as possible. On the societal side, using facial recognition technology is heavily debated. We also need to be careful that solutions built with a certain intent in mind are not repurposed for other purposes, and set strong boundaries on the use of the application.
7. Accountability:
The final dimension, Accountability, is about assuming responsibility for actions and decisions. We need to be able to identify if harm was caused and know how to rectify potential negative effects.
- Who is responsible if users are harmed by the AI solution?
- Who will have the power to decide on new features or changes to the system?
- How do we keep track of and document decisions after launch (e.g. expansion of use, changes in access, etc.)?
Accountability is in our case not one of the highest risk dimensions. In our experimental case, the responsibilities can be quite clearly assigned: the ML engineers built an original solution and could be responsible for monitoring and documenting decisions after launch. If the solution was actually implemented in our office, management should be accountable for any decisions, changes or actions taken related to the solution. Of course we should set boundaries on how long the solution will be in use (e.g. until regulation is lifted), and on how the tool is allowed to evolve in the future (e.g., requirement to stay on edge).
As we can see, the main ethical risks in this use case lie in the dimensions Privacy and Data governance, Diversity and Fairness, and Technical Robustness - let’s call those the high risk dimensions. For each dimension, we need to ask ourselves what we could do to mitigate the risks. Starting off, we will need to inform everyone visiting our office on what the solution does and how it works (how accurate it is, which technology it’s using, etc.) and why we use it. On the privacy side, we might want to check the possibility of anonymising faces on edge, which might be a trade-off with performance. We might also consider giving individuals the possibility to opt-in/out of the solution and need to make sure that no one could get (and implement) the idea of saving personal data generated. Regarding fairness, we could assign one of our engineers to assess the solution for bias regularly. On the robustness side, we would need to monitor the solution in use and improve its robustness if we realize that it’s being tricked, as well as make the solution as robust as possible against potential hacking attacks. Last but not least, we need to set clear boundaries for the solution - deciding on when we’d stop using it (latest when health regulations are lifted). These are just a few ideas of mitigating actions, of course the possibilities are broad and depend a lot on the solution and the context.
In a real AI Ethical Risk Assessment, we would now guide our clients through the decision process - do the benefits outweigh the risks? Which mitigating actions do we need to implement to make sure we are building a Trustworthy solution? Real world use cases can of course get much more complex than the simplified hypothetical example we described - an Ethical Risk Assessment can be a good starting point to think through the risks of an AI solution systematically, and get prepared for the upcoming regulation by the EU.
Do not hesitate to reach out if you have any questions or are curious to know more!
Looking for more inspiration on questions to ask in the different dimensions? Check out for example the Tarot Cards of Tech and the Assessment List for Trustworthy AI.
No items found.