April 28, 2021
Pharma 4.0 : Impact drug manufacturing with AI in Life Science Industries

In this blog, we will uncover some pressing challenges within the Life Science manufacturing industry and how breakthroughs in Machine Learning techniques offer measurable returns.
From batch to continuous manufacturing and pharma 4.0
Pharmaceutical companies are often in a race against time. Although patents protect companies intellectual property, most of this time is spent turning an idea into a marketable product. Traditionally medicines are produced in the old-fashioned way by a batch process [3]. This traditional batch process has proven to have high lead times, because after each process step the production is stopped to test for quality assurance. Sometimes these materials are stored in containers or shipped to other facilities before they continue in the process [5]. Each stop increases the lead time and can cause defects and scrap [6]. As an indication, lead times can be up to 365 days of which 228 are dedicated to drug substance production, 75 to drug product formulation and 41 to packaging. Inventories including raw-material storage can last 250 days [1]. Reducing these times is essential to recover the billions spent in drug development given the fact that there only a few years left before the patents are expired.
The pharmaceutical industry is often compared to the semiconductor industry due to the high costs and the need for high throughput, volume and yield in a clean environment with high consistency [2]. The semiconductor industry is already quite matured when it comes to implementing industry 4.0 and this has resulted in major advancement in technology (i.e. smaller chips with greater capabilities in computers, phones, etc.). But what is the state of Pharma 4.0? How is this industry moving towards the future? When compared to the semiconductor industry there is a difference in the demanding regulations enforced by authorities like the US FDA and the EU Commission to ensure the quality. Changes to production in the form of digitization can result in changes to machines, processes and even the product itself. It is these strict regulations which are a possible cause of the conservative character of the industry when compared to the semiconductor industry.
Today, next to the stringent manufacturing requirements, the industry is entering an era of smaller batches and personalized medicine. Medicine is designed with more unique features and needs to be delivered quicker to patients in need [6]. In other words, drug production requires very small batches often measuring in sub-liters tuned to individualized genomes. [1].
To allow smaller batches and more cost effective production of drugs, the industry is changing to continuous flow manufacturing as shown in Figure 1. Small amounts of chemical ingredients flow without disruption from raw ingredients to tablet. In 2016 the FDA encouraged manufacturers to transition from batch to continuous manufacturing due to its many benefits [6]. This new method has the potential to cut drug manufacturing times by 90% and costs by 30-50% according to Novartis [9] or gain an additional $50 billion in annual revenue [1]. However, it required to have a seamless process integration and full process control, which can be achieved by operational data and automation. Leading pharma giants have already been working for multiple years on continuous manufacturing e.g. Johnson & Johnson Janssen won the FDA’s approval to switch from batch to continuous manufacturing [10] and Novartis entered a 10-year research collaboration program with the Massachusetts Institute of Technology (MIT) in 2007 [11].

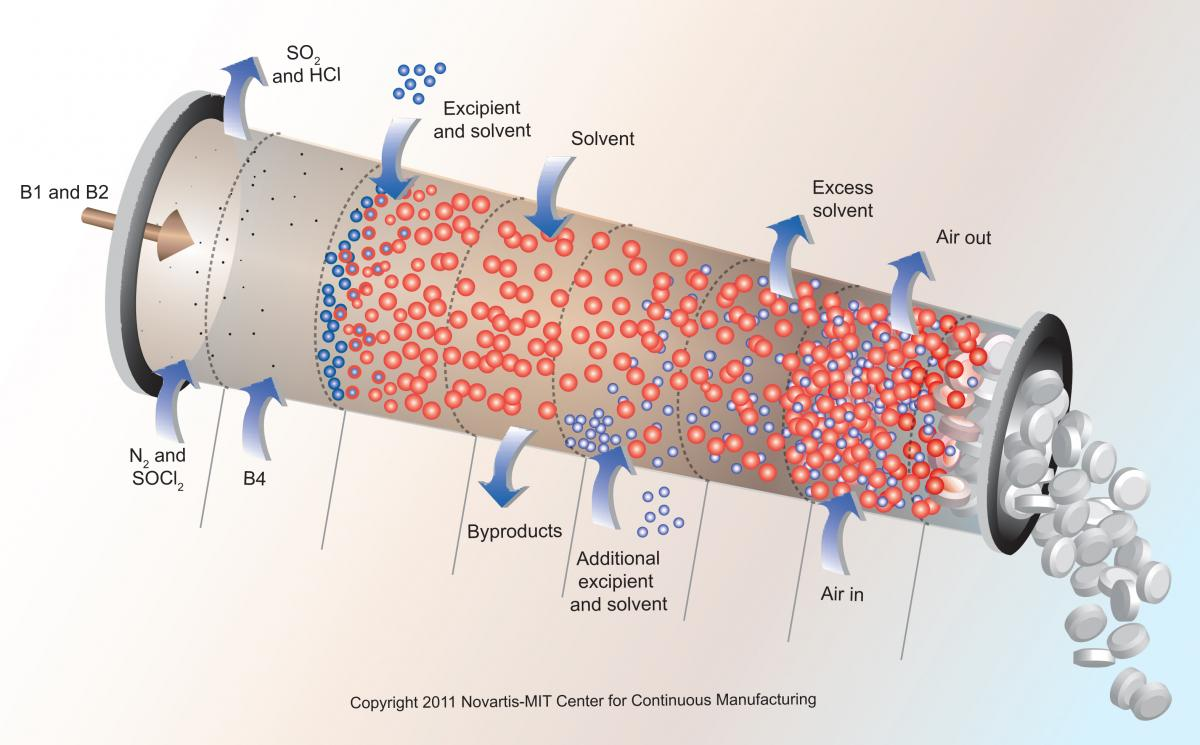
So how can AI help with drug manufacturing?
No matter if you have a batch process or a continuous manufacturing process, AI projects require data and a process which is repetitive, has a measurable outcome and a certain uncertainty in order to be successful, as discussed in ML6s blog on how to boost your manufacturing process with AI [12]. Data can be in several forms, from microscopic lab data, to visual inspection data to IOT data gathered from the machine and visualized real-time in dashboards. Tips and tricks on the latter can be found in the following blogs [13] and [14].
As discussed above, continuous manufacturing processes require ultimate process and quality control. One of the advantages AI can offer is connecting the sensor data to lab results as depicted in Figure 2. Thanks to the use of open standards, such as OPC-UA on PLCs, incorporating machines and lines from different brands allows for easy access to your machine data. The data can be stored in a suitable database such as InfluxDB or Prometheus. Instead of manually testing a few samples per X hours, predictive algorithms can predict lab-results using real-time data for every single batch or drug.
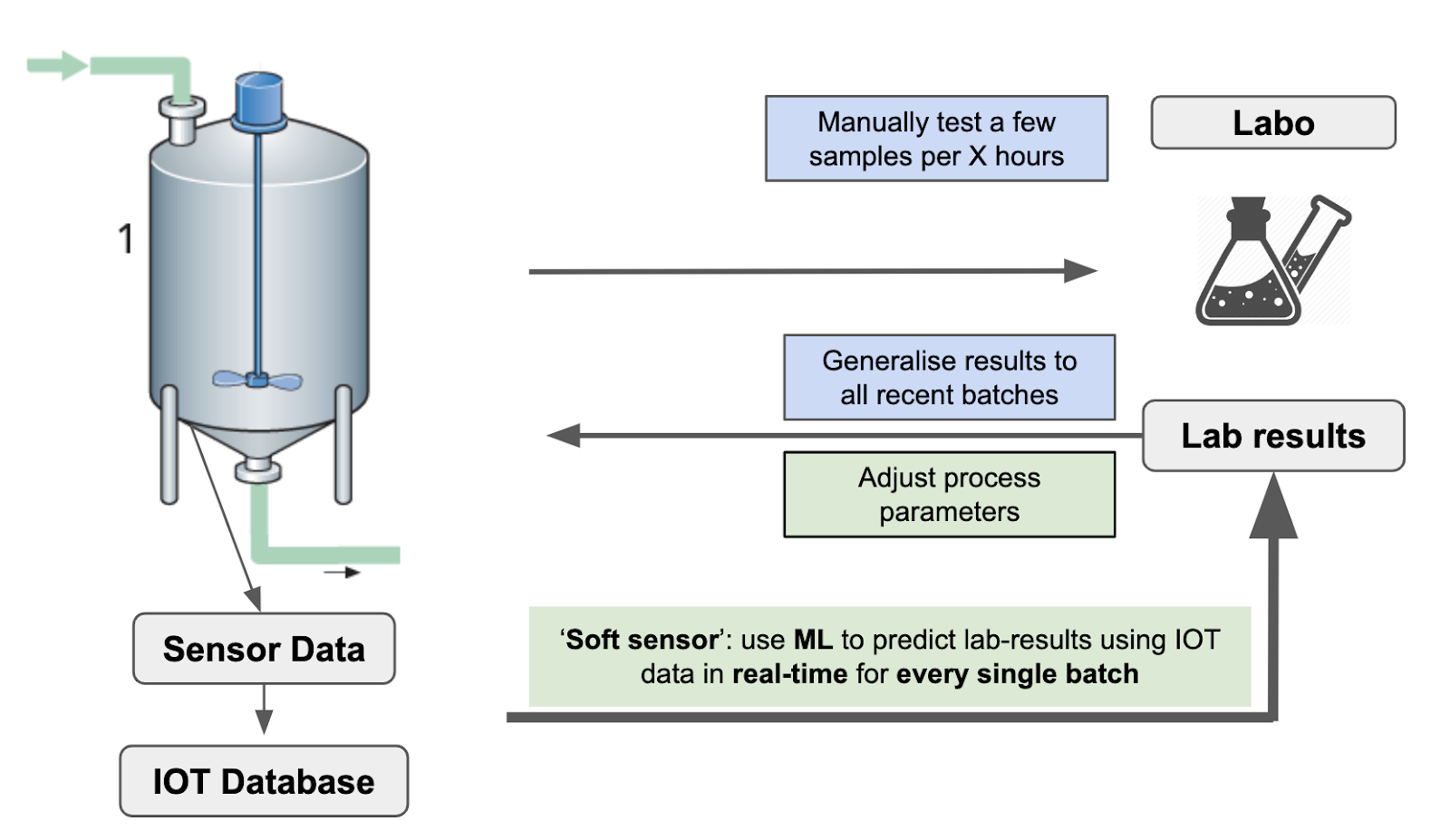
Next to quality control by IOT data, one can use machine learning algorithms to automate visual inspection of medicine foil strips checking for container and closure, information which is written on the label (such as brand name, ingredient names, manufacturer name and logo, batch/lot number, expire data etc.), and physical characteristics of the tablets/capsules (such as uniformity of shape, size, colour, texture, breaks, cracks, splits, markings, empty capsules etc.). Even on a microscopic scale you can e.g. classify microorganisms in Pharmaceutical Microbiology or particle size detection. A common technique used in Machine Learning is image segmentation to distinguish common objects or microscopic particles as shown in Figure 3.


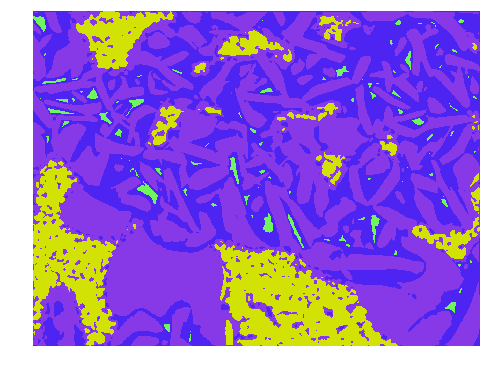
The first step of improving efficiencies is to create insight in your manufacturing machine data. An example is the Bosch Pharma i 4.0 Starter Edition [17] which offers condition monitoring, event tracking and measuring Overall Equipment Effectiveness (OEE). Similar dashboards can be created customized using open source technologies such as InfluxDB and Grafana [13]. Historical data provides the possibility to do anomaly detection or predictive maintenance. Note that analysis on track and trace data for traceability purposes can also be performed to make sure you are fully compliant to regulations. Next predictive algorithms can be developed to create foresight to operators or higher management.
Countless parameters in a manufacturing process can be mapped and optimized. Doing this manually is a tedious job and basically impossible due to the amount of possibilities and varying conditions. Using machine learning, you can implement a full autonomous parameter optimizer using a self-learning system to find the optimal solution in every circumstance to increase efficiency. Sample use cases are the improvement of a powertrain on an electrical bike and reducing the energy consumption of a datacenter, and this can be applied to drug manufacturing as well [12]. Improving production, quality and safety days while reducing consumption of raw materials. A schematic outline is shown in Figure 4 of such a self-learning algorithm.

Medicine often have different lead times and specific transport or storage requirements. Specific requirements are: drugs which require temperature control resulting in limited shelf life; flammable or explosive drugs which need to be handled carefully; narcotics or psychotropic drugs which require close monitoring due to stringent regulations etc. These requirements can cause complexities when it comes to storing and transportation or finding the optimal planning and demand forecasting. Using machine learning techniques one can optimize the planning and forecasting both inside and outside manufacturing facilities. An example of a self-learning algorithm can be found in Figure 5 where the optimal route is found for a single driver in an environment with no obstacles (left) or a multi driver optimization problem with multiple obstacles. The transport/storage requirements act as the obstacles in this example. Note that similar AI techniques can be used for demand forecasting in your supply chain.

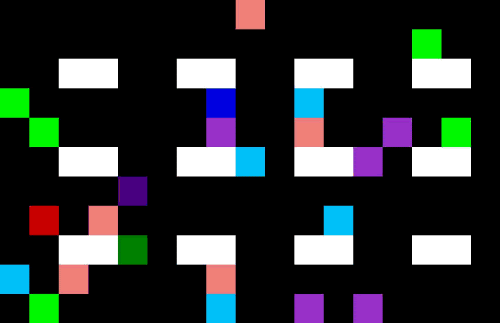
More information on how to apply AI in drug manufacturing can be found in this video.
On a final note...
To enhance time to market and quicken development time of AI solutions, a comparison can be made with the semiconductor industry where “ASML boosts engineering speed, strengthens security, accelerates time to market, and enhances competitive advantage by adding Google Cloud to its on-premises machine learning solutions”. Examples like these show how the pharmaceutical industry can catch up on the semiconductor industry and move to the industry 4.0 which can result in great technological achievements, increased profit and reduced time to markets.
No items found.