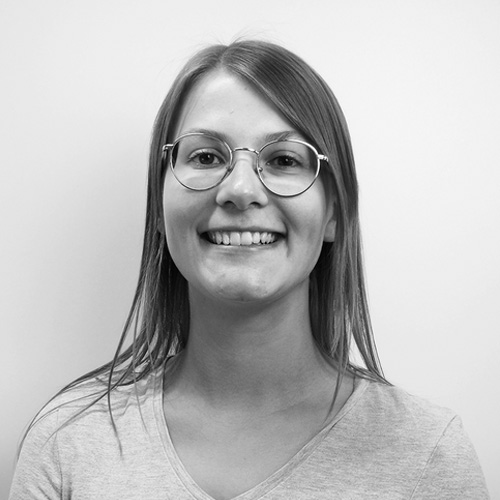
Pauline Nissen
Ethical AI Lead
In this article, we explore how to navigate the requirements for developing high-risk AI systems within the regulation of the European AI Act, ensuring compliance and fostering ethical AI innovation.
If you’re immersed in the world of AI, the recent developments around the European AI Act are likely on your radar. If not, it’s the right time to proactively think about the impact of the AI Act on your (future) AI project. The AI Act, adopted after extensive discussions among the EU institutions, marks a significant move towards regulating AI technologies across the European Union to balance citizen protection and technological advancement.
If you’re new to the AI Act, let’s review the key points you need to understand. If you are already the AI Act expert of your company, feel free to skip ahead to the next paragraph.
In short, the AI Act is the first European-level regulation specifically aimed at overseeing AI. The journey to adopting the AI Act involved deliberations, negotiations, and revisions, resulting in a political agreement in December 2023. While a draft version of the AI Act is already available, the final text is expected to be published in the coming months. Once entered into force, there will be a transition period of 24 months before the provisions become fully applicable, with certain exceptions taking effect sooner at 6, 12, or later at 36 months.
At its core, the AI Act uses a risk-based approach, classifying AI systems into different risk levels based on their potential impacts on individuals and society. For instance, some AI systems pose too many risks and are consequently prohibited (think about manipulative or very intrusive systems). Other AI systems present high risks from an ethical perspective. For example, if the systems are not properly designed and developed, they could potentially lead to discrimination, violations of fundamental rights, or impact society or someone's right to privacy in a negative way. These AI systems are not prohibited per se, but measures should be implemented to identify and mitigate those risks. Then, there are AI systems with lower risks that still require specific measures to ensure transparency.
As indicated in the title, this article will focus on high-risk AI systems and how to navigate the necessary compliance requirements. If you're unsure whether your (future) AI project falls into the high-risk category, we will soon release another blog post about the classification of the AI systems under the light of the AI Act.
You might wonder why you should consider the AI Act now when most provisions won't be applicable for one or two years. At ML6, we see 3 main perspectives to consider:
From an ethical standpoint, adhering to the AI Act early on enables the development of responsible AI solutions. Viewing the AI Act just as an administrative burden overlooks its benefits. Proactively identifying and mitigating risks enhances the quality of your AI system. For example, this proactive approach helps identify and reduce biases to create a more fair AI solution prior to its development or its production release. The requirements also emphasise security best practices and transparency, fostering user trust in AI systems.
From a legal and financial standpoint, non-compliance with the AI Act, especially for high-risk AI systems, can lead to substantial fines - up to 35 million euros or 7% of your company's global revenue for major violations. Even minor infractions can result in fines of up to 7.5 million euros or 1.5% of turnover. Next to fines, non-compliance with the AI Act could lead to liability if the system would cause damages to individuals. This situation reminds us of what happened with GDPR. Based on past lessons, we suggest getting ready for the AI Act before it becomes too urgent.
From a technical viewpoint, developing AI solutions is a time-intensive process. If you understand the AI Act requirements early on, you can integrate them into your project from the beginning. This makes compliance easier later and prevents the need to overhaul your technical setup or write extensive documentation when your AI system has already been developed two years ago. At ML6, if we identify that a client’s project falls into the high-risk category, we inform and advise our client about the upcoming requirements. Our way of working also allows us to implement these requirements early on, such as conducting risk assessments, adopting a security-by-design approach, and closely collaborating with users throughout the development.
So what should you do if your AI project falls into the high-risk category? In this paragraph, we provide our general impressions of the requirements, go deeper into the specifics of these requirements, and share our experience on proactively implementing them.
Chapter 2 of the current AI Act version describes the seven requirements for high-risk AI systems. Our general impression is that, while the legal aspects of the AI Act have been well-considered, the precise technical implementation of these requirements remains somewhat unclear. Terms like "judged to be acceptable", "as far as technically feasible" and "where appropriate" suggest that further clarification and practical guidelines will be necessary to minimise subjective interpretation. Another observation is that most requirements focus on enhancing transparency in AI systems, such as documenting the development process, providing user instructions, and implementing logging mechanisms to record AI system outputs. Transparency plays an important role in building trust in AI among individuals and society. Additionally, the extensive documentation and logging requirements also serve the purpose of enabling authorities to verify compliance with the regulation.
1. Risk Management System
To fulfil the first requirement on risk management system, you need to establish a continuous and iterative process for identifying and mitigating risks associated with your project. This involves identifying foreseeable risks under intended use and potential misuse scenarios, implementing risk management measures, and testing the effectiveness of these measures. The continuous and iterative nature of this requirement is very important. You can start with an initial risk assessment analysis at the start of your AI project using frameworks such as the Assessment List for Trustworthy AI. However, it’s important to re-evaluate those assessments if there are any changes in the project scope, such as adding new data sources or involving users with diverse backgrounds or interests. Throughout the development lifecycle, it’s also important to pay attention to new insights that may impact the risks analysis, as discoveries during the development process may reveal additional non-identified risks that you need to address.
2. Data governance
The second requirement of the AI Act focuses on data governance, emphasising the importance of high-quality data when developing AI applications. But what exactly does "high-quality data" mean? It means ensuring that your training, test, and validation datasets are relevant, representative, complete, and as error-free and unbiased as possible. To achieve this, there are several data governance best practices to follow. For instance, to mitigate biases in your dataset, start by understanding the business context and analyse your data for biases. Techniques like creating a balanced dataset can help reduce bias. In the context of the AI Act, it's important to document your data governance practices, including data preparation, validation, and monitoring steps.
3. Technical documentation
As mentioned in the previous paragraph, documentation plays an important role in the light of the AI Act. Documentation not only promotes transparency but ensures compliance with regulatory requirements. In Annex 4 of the AI Act, you will find a detailed list of information that must be included in your technical documentation, covering aspects such as the AI system's goals, architecture, capabilities, limitations, and processes. If you're dealing with multiple high-risk AI systems or plan to do so, we strongly recommend creating templates with predefined sections outlining the required information. It's important to note that while AI developers often write the technical documentation, interpreting and implementing AI Act requirements may benefit from legal expertise. Consider seeking external advice to effectively translate regulatory requirements into practice.
4. Record-keeping
With record-keeping, or what we also call logging, you need to automatically record events of your AI systems to ensure traceability. Those logs can be used to identify potential risks and to monitor the system once it has been released into production. By leveraging detailed logs, you can effectively track system behaviour, diagnose issues, and maintain accountability throughout the lifecycle of your AI system.
5. Transparency
Transparency in AI involves ensuring that users understand the capabilities and limitations of the system. This means providing clear details and instructions on how to use the AI effectively. By doing so, users can make informed decisions and use AI responsibly. Our recommendation is to directly engage with users during the development process of your AI system. Consider organising training sessions to explain how the system works, potential risks like overreliance or bias, and how to handle any issues that come up during use. Offering a basic introduction to AI can also help users feel more comfortable and confident using the technology responsibly.
6. Human oversight
Human oversight involves ensuring that natural persons have control and oversight when needed. This means having a responsible individual who ensures that the system functions correctly as intended and can intervene to prevent unintended actions. It's important to assign this role to someone with the appropriate responsibilities and training to understand the AI system's limitations and know when intervention is necessary.
7. Accuracy and cybersecurity
Accuracy and cybersecurity are important aspects concerning the performance and security of AI systems. Using metrics to measure performance ensures that the AI system works as intended, although accuracy alone shouldn't be the only metric used. It's important to consider a range of metrics to get a full picture of performance (think about fairness metrics). In addition to performance, you need to protect your system against different types of attacks. This includes safeguarding against common software attacks and specific vulnerabilities such as prompt injections that can affect AI systems.
In wrapping up, the AI Act marks a significant milestone as the first European-wide regulation designed to oversee AI technologies. This legislation adopts a risk-based approach, classifying AI systems according to their impact on individuals and society. Even though the AI Act won't come into full effect for a while, it's wise to start familiarising yourself with the AI Act, its workings, and the specific requirements for high-risk AI systems. An important focus of the legislation is around transparency, aiming to build trust between individuals and AI systems. Although certain aspects of the law require further clarification, gaining an initial understanding now will not only help mitigate the risk of fines but also enable the development of responsible AI solutions and prevent the need for extensive project modifications once the AI Act is fully enforceable.