December 1, 2022
AI for the green transition - Applications and use cases [Part 1]
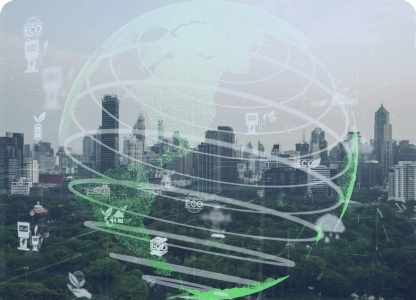
AI has great potential to accelerate efforts to protect our environment, such as reducing emissions or making more efficient use of scarce resources. Let’s dive into some examples of use cases - with no ambition to be exhaustive - where AI can help tackle important challenges.
In a series of blogposts, we will look at 4 dimensions, in line with the EU Green Deal actions, covered in the visual below. In this first blogpost, we will deepdive into the topics of climate action and clean energy and sustainable industry and Agriculture.
.png)
Climate action and clean energy
The European Green Deal aims to make Europe climate neutral by 2050 and has set ambitious greenhouse gas emission reduction targets. This can only be achieved if emissions are reduced in all sectors. Energy especially is critical to reaching the targets, given that more than 75% of EU greenhouse gas emissions stem from the production and use of energy. AI technology has already seen applications to help these efforts, such as:
Measuring and predicting greenhouse gas emissions
Companies face increasing pressure to transparently, accurately and frequently report emissions and take actions to reduce them. Precise measurement on a granular level (e.g. product or process level) is key to know where to focus efforts; however, manually measuring emissions is time-consuming, data-intensive and costly. AI and data analytics can help to effectively track emissions and identify inefficiencies, improvements and opportunities for lower emissions. Consider calculating the supply chain carbon footprint based on invoices and emission data to allow real-time emissions tracking (e.g. Snowfox). Another example could be an AI-enabled platform, such as CO2 AI by BCG, to measure and reduce emissions across the value chain and collaborate with suppliers. Using AI to predict future emissions can also help to adjust and achieve emission reduction targets.
Improving energy efficiency and reducing consumption
Predictive capabilities can predict and manage energy demand and supply, increase operational efficiency, coordinate decentralised energy networks, and enable faster control and more flexible adjustments to unforeseen events. The latter two examples will gain even more importance with the move towards renewable energy, which is more prone to uncertainties and fluctuations (e.g. due to weather conditions) than sources such as gas or coal. On the consumption side, AI can help study energy consumption patterns and use those insights to help save energy. For example, the heating and cooling of buildings can be adjusted more flexibly based on data such as weather conditions and sensors capturing occupants' habits. In the public sector, ML6 is currently working with the Agency for Roads and Traffic (AWV) to reduce energy consumption by street lights, using real-time data on traffic intensity and weather conditions to control road lighting more accurately and more location-bound.
Transitioning towards renewable energy
AI can enable renewable energy sources to become more cost-effective, efficient or predictable, helping to shift the energy mix more towards renewable, less carbon-intensive energy sources. For example, ML6 worked with the Belgian wind farm provider Otary. With the help of ML technology and explainability techniques, we predicted the root causes of energy underproduction, taking into account sensor data such as wind direction, turbine location, and weather conditions. These insights enabled the wind farm to find the driving factors leading to underperformance and ultimately decide which actions to take to generate more green electricity from their wind farms. Similarly, the positioning of solar panels could be monitored and adjusted based on local weather to maximise power production.
Sustainable Industry & Agriculture
We can only achieve a circular and climate neutral economy through the mobilisation of industry, which needs to become greener, more circular and more digital (EU Industrial Strategy). Similarly, a sustainable EU agriculture and food system benefits the environment, health and society. In both areas, AI technology is already being used, for example in applications such as:
Reduction of waste and defective production
Reducing the number of defective products and waste in the manufacturing process can reduce raw material consumption and save energy. AI-enabled quality control systems based on computer vision on the production line, an area where ML6 has conducted many use cases in recent years, can help reduce production defects and waste and increase the efficiency of the process. Using sensor data during production can be another way to identify bottlenecks and reduce waste. For example, ML6 worked with Accolade wines, the largest wine manufacturer in the UK, to help them save 1 million litres of wine per year by identifying when wine loss occurs and alert operators to investigate and take instant action.
AI for smart logistics and stock management
Better forecasting of demand can help to manage stocks better and optimise the design of logistics strategies. Excess products not only waste resources through their production but also cause greenhouse gas emissions when shipped and stored in climate-controlled warehouses. By implementing demand forecasting based on this available data, supply and demand will be better balanced, lowering the produced waste. This avoids sunk costs for the company while at the same time reducing its carbon footprint. Also, AI can be used to make distribution and logistics operations more sustainable, for example, by optimization of routes for delivery.
Precision agriculture
Precise monitoring and managing environmental conditions in agriculture with the help of AI can reduce the need for pesticides and fertilisers, reduce waste and use of water, and increase output. Field sensors can be used to measure various factors that impact agriculture, such as local weather, to predict the impact on yield and required measures. For example, pests can be identified and monitored to predict the optimal (minimal) use of pesticides needed. Farmers can also monitor crop health to inform better management and take action. This can lead to higher yield and lower cost base for producers, and more sustainable agriculture for the environment.
In the agricultural sector, ML6 worked with CNHi Industrial on an AI solution for their new line of combines. These combines are equipped with a camera and hardware. The goal is to detect the quality of the crop that is being harvested by running image segmentation on the hardware and images taken by the camera. Based on this information, the combine can alter its parameters accordingly.
You can access our second blogpost where we deepdive into the topics of transport and mobility and environment and oceans here.
No items found.